
The team used tiny sensors implanted on the participant’s brain to read the intent to move, allowing them to track and analyse brain activity despite the participant being unable to physically move.
| Photo Credit: Special Arrangement
Researchers at the University of California, San Francisco (UCSF) have achieved a significant breakthrough in assistive technology for individuals with paralysis. The lead author of a scientific paper on the project, an Indian who grew up in Chennai, Nikhilesh Natraj, says they have developed a brain-computer interface (BCI) that allows a paralysed man to control a robotic arm simply by imagining the movements he wishes to execute.
Dr. Natraj is a neuroscientist and neural engineer at the Weill Institute for Neurosciences, UCSF. “Here, our team has developed a framework that allows a paralysed man to control a robotic arm for 7 months straight using just his thoughts alone, with minimal calibration,” he says. The results of this study were published in a recent volume of the peer-reviewed journal Cell.
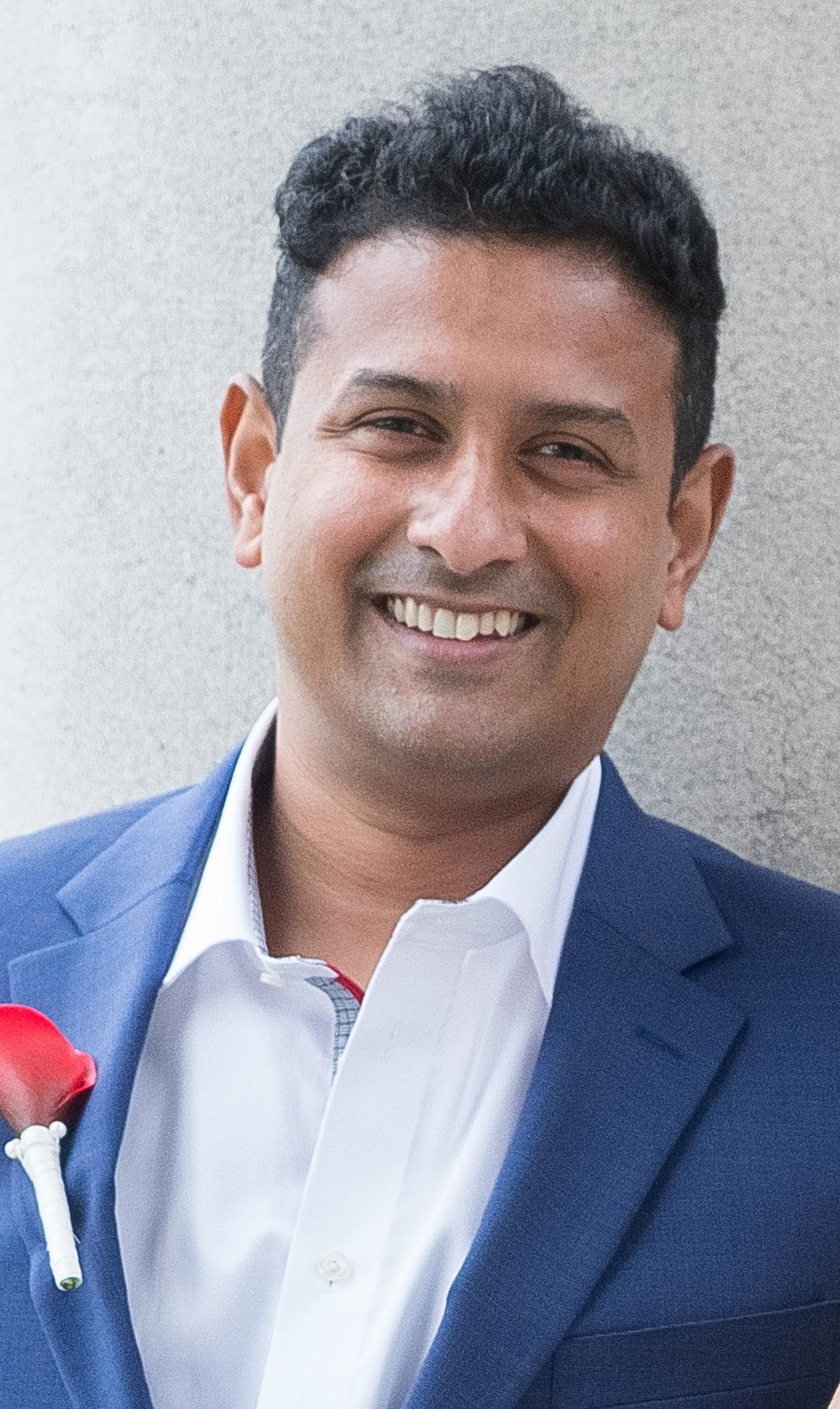
Dr. Natraj and his team developed a framework that enabled a paralysed individual to control a robotic arm using only his thoughts for 7 months, with minimal calibration, showcasing the potential of BCIs for long-term, stable use.
| Photo Credit:
Special Arrangement
Developing stability in Brain-Computer Interfaces (BCIs)
To start with, the team had to understand the neural patterns behind movement. The key was discovering how activity shifts in the brain day to day as a study participant repeatedly imagined making specific movements. Once a machine learning/AI algorithm was programmed to account for those shifts, it worked for months at a time.
Karunesh Ganguly, professor of neurology and a member of the UCSF Weill Institute for Neurosciences who studied how patterns of brain activity in animals represent specific movements, saw that these patterns changed day-to-day. If one assumed that the same thing was happening in humans, these changes would explain why the participants’ BCIs became unstable and quickly lost the ability to recognise movement patterns. The team worked with an individual who had been paralysed by a stroke and could not speak or move, a note on the varsity website stated.
The study participant had tiny sensors implanted on the surface of his brain that could pick up brain activity when he imagined moving. The sensors do not send pulses to the brain, but only read out the intent to move from the movement regions of the brain, Dr. Natraj explains.

AI and signal processing
To see whether and how his brain patterns changed over time, the participant was asked to imagine moving different parts of his body. Although he couldn’t actually move, the participant’s brain could still produce the signals for a movement when he imagined himself doing it. The BCI recorded the brain’s representations of these movements through the sensors.
Analysing the patterns in the high-dimensional sensor data, the team found that while the structure of movement representations stayed the same, their locations in the high-dimensional data shifted slightly from day to day. By tracking these shifts and predicting how it would evolve, the team was able to overcome instability in BCI systems and developed an end-to-end signal processing and AI framework.

From imagined movements to real-world actions
The participant was then tasked with imagining himself making simple movements with his fingers, hands or thumbs while the sensors recorded his brain activity to train the AI. The read out signals were then decoded to actuate a robotic arm. Initially, he practiced on a virtual robot arm that gave him crucial feedback on the accuracy of his visualisations, helping him refine his direction and control.
Eventually, the participant managed to control a real-world robotic arm executing the action. He could perform tasks such as picking up and manipulating blocks, turning them, and relocating them. He even managed to open a cabinet, retrieve a cup, and hold it under a water dispenser—simple tasks but those that can be life-changing for those living with paralysis.
Having established that it can be done is the first stage, a lot more work needs to be put into refining the technique and for it to be deployed among people who have paralysis, Dr. Natraj says. Especially, the system should be able to work fluidly in complex scenarios with many distractions, such as when going to a crowded grocery store, he adds.
Published – April 28, 2025 07:06 pm IST